Is Responsible AI MLOps in Disguise?

The promise of generative AI has been laboriously discussed by everyone riding the Gartner hype wave, and while the height of the high water mark may not yet be fully visible, there is building consensus that public services stand to benefit greatly from its transformative potential. Generative AI, and its now-not-as-exciting predecessor, machine learning, will be critical to meet the rapidly evolving needs of the population - from automated but personalised communication, faster emergency responses, to entirely new creative solutions to existing problems. Personally, I can’t wait to finally speak to chickens.
Whatever the use case, it is paramount that this new technology is used responsibly, to ensure that AI-powered services are fair, transparent, and accountable, building trust with citizens to deliver positive societal outcomes.
There is no way around it - the term ‘responsible AI’, while not entirely new, is becoming the latest ubiquitous buzzword, often used without clear and consistent definition, much like “disruption-free innovation” or “synergy-driven collaboration”. Everyone agrees that it’s important we do it, but the lack of a unified understanding can make it feel challenging to translate the concept into concrete actions.
Last year, Databricks published its 6 principles for responsible AI (generative or otherwise). Many of the points made in the article centre around strong foundations in data, governance and control, which raises the question: can we adapt existing best practices in data and AI management to generative AI to succeed in this new world with confidence?
In the next few hundred words I’ll argue that Responsible AI isn’t just a fancy term – it’s the practical application of already-established MLOps principles to the unique challenges of generative models, and briefly explore how organisations can take the lessons learned from working with the principles of MLOps into the relatively new discipline of generative AI.
Building on a Solid Foundation
MLOps is broadly understood to be the Shelley-esque combination of model, data and DevOps dev ops, and their integration within the broader organisation. I won’t say that generative AI doesn’t present unique challenges, but it shares a startlingly significant amount of common ground with traditional MLOps, even if these may not yet be universally understood.
MLOps emphasises the need for strong technical foundations, with robust quality assessment, monitoring, version control, and lineage tracking for models, the code that produces/deploys them, and the data they consume at various stages of their lifecycle. This is no different in the world of generative AI, where managing models alongside code and data is key for trusted and reliable AI systems.
These technical foundations don’t exist in a vacuum. MLOps encourages the definition and implementation of operating models to manage this technical lifecycle effectively. From identifying and tracking business needs, through strong technical personnel for building models, to approving deployment and reviewing decisions, human oversight is at the core of MLOps and responsible AI alike.Managing societal impact is a critical aspect of responsible AI. Understanding and mitigating bias, harm and a lack of equality and fairness in models is paramount in (and out of) the public services. The foundations of human-in-the-loop, monitoring, controlled experimentation, and more within MLOps serve as the basis for minimising these adverse and unintended effects. Although this is often still work in progress even for traditional ML, there are many lessons, such as policy-driven postprocessing of outputs or continuous learning designs, that may be learned from MLOps to be carried over to generative AI.
Lastly, and perhaps most importantly, the central tenet of MLOps is continuous improvement. Machine learning models are living and breathing beings, and between continuous experimentation, use case definition and re-definition, and quality monitoring managing the lifecycle of these models is never done. This is no different with generative models, where the true and useful integration of them will mean welcoming them into existing delivery cycles.
Recognising these shared principles of technical foundations, operating models, societal considerations and continuous improvement will give organisations a head start in understanding and build confidence in deploying and leveraging generative AI.
Beyond a Simple MLOps Copy-Paste
Look, I’m acutely aware that generative AI presents entirely new challenges, and requires new skills and technologies - put down the pitchforks, all ye Prompt Engineers and Generative Design Specialists.
Most obviously, generative models are… well, generative. Whereas the possible outputs of a binary classification model are very well defined, it is hard to understand and control the full scale of things as ChatGPT might say. This means the potential for unexpected or harmful outputs is higher, requiring a more nuanced approach to monitoring and risk mitigation.
If predicting the things gen AI might do or say is difficult, explaining how or why they do or say those things is yet another category of complication. Grappling with interpreting model weights in traditional settings is challenging enough, doing the same with even the smaller LLMs will be a mountain task. Here, emerging techniques like inter-communicating agent systems might hold promise. These systems allow models to "explain" their decisions by interacting with each other, offering insights beyond simply analysing the internal workings of a single model.
The increasing use of generative models as APIs, rather than internally managed objects, presents a new challenge. While monitoring remains crucial, directly influencing the performance of these external models might be limited. This necessitates robust collaboration and clear service-level agreements with API providers to ensure responsible AI practices throughout the chain, taking the reach of end-to-end MLOps outside the organisation.
Taking Steps to Bridge the Gap
It’s not all doom and gloom, however. I’m a firm believer that, no less than any other industry, the public sector will unlock the full power of generative AI by extending, and not replacing, their established MLOps practices:
Evolution, not Revolution – embrace a mindset of evolving your current MLOps strategy to encompass generative AI. Leverage existing data governance, access controls, and audit trails while incorporating techniques like inter-agent communication for explainability.
Holistic Product View – shift from siloed model development to treating AI systems as complete products, with comprehensive risk assessment that goes beyond technical performance, and considers the business impact, associated risks and ownership, ensuring responsible deployment from conception to real-world use.
Human-Centred Development – maintain a clear path to production with well-defined steps for human oversight and intervention. Integrate human expertise into the decision-making process, particularly when dealing with high-stakes outputs. This human-centred approach fosters trust and ensures AI serves the public good.
Building Trust Through Action
Generative AI offers a transformative toolkit for the public sector, but trust from its creators and consumers alike is paramount. Building upon, not abandoning, established MLOps principles is the key. Recognising shared focus on technical foundations, operating models, societal considerations, and continuous improvement allows organisations to confidently leverage generative AI.
However, a simple copy-paste of MLOps practices won't do. The inherent generative nature demands a nuanced approach to monitoring, risk mitigation and explainability. Collaboration with API providers and fostering human-centred development are crucial for ensuring responsible AI throughout its lifecycle.
The public sector can unlock the full potential of generative AI by embracing an evolutionary mindset, treating AI systems as holistic products, and prioritising human oversight. This action-oriented approach builds trust and ensures AI serves the public good, delivering on the transformative promises it holds.
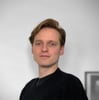