How can AI be deployed ethically in the public sector?

The age of artificial intelligence (AI) is here and changing things more rapidly than almost any technology in history. It promises to drive efficiencies and enhance services, but the rapid speed at which it is being embedded in the public sector has raised awareness about the ethical implications of implementing these technologies.
Left unchecked, it presents serious risks to citizens. An AI weapon scanner used in hundreds of US schools failed to detect almost 50% of knives. Another scandal in the Netherlands, where machine learning was used to detect welfare fraud, falsely accused thousands of parents of child benefits fraud.
Clearly, AI can be a powerful innovation tool - but only if it is implemented ethically and safely. A group of experts from the Centre for Data Ethics and Innovation (CDEI), Faculty AI, Innovate UK and the academic community share how they are mitigating the risks - and the ongoing challenges involved in getting this right.
What does good look like?
The Centre for Data Ethics and Innovation (CDEI) was set up to promote responsible innovation of data-driven technologies. The centre, which is part of the Department for Science, Innovation and Technology Department, works with private and public bodies that are building AI or data-driven products, or are seeking to regulate or promote innovation in those areas.
Felicity Burch, Executive Director at the Centre for Data Ethics and Innovation (CDEI) says when it comes to AI, it is important to know exactly what's in the box. She believes AI deployment represents a “huge opportunity” for the public sector, but admits there is a need for more education around the ethical risks associated with this technology and how to address them. “The more you get to the AI technologies at the frontier where people have less experience, the more those questions rise to the fore. One association with AI we often hear is snake oil: organisations don't really know what vendors are selling.”
CDEI is focused on giving organisations the tools they need to navigate this landscape. It developed one of the world’s first algorithmic transparency standards to encourage organisations to disclose to the public information about their use of AI tools and how they work. “We are currently focused on making this more widely available and easier for people to use,” Burch says.
A crucial factor is assessing and demonstrating the trustworthiness of AI tools, Burch notes. But a recent survey reveals that organisations do not always have the skills or knowledge to do this. “The challenge we've got back from industry is they want to know what good looks like. We found that organisations don’t know what assurance techniques exist, or how these might be applied in practice across different contexts and use cases.”
In response, CDEI has published a portfolio of AI Assurance Techniques, which showcases examples of AI techniques being used in the real-world. The portfolio aims to give those involved in designing, deploying or procuring AI tools examples of assurance practice for the first time.
Burch says CDEI’s latest push to address bias and discrimination across the AI lifecycle is a Fairness Innovation Challenge; designed to help organisations measure whether algorithms are fair or not. “Defining fairness and measuring algorithmic fairness is quite hard,” Burch explains. “Regulators and other organisations have questions about how best to approach this.”
CDEI is currently undertaking a call for use-cases to unpack what the main challenges are; this could be concerns around demographic data or the quality of the analysis required. The long-term aim is to work with regulators to eventually develop a solution, Burch says.
Fostering transparency and trust
Albert Sanchez-Graells is a Professor of Economic Law at the University of Bristol Law School and Co-Director for the Centre for Global Law and Innovation. He believes the UK government’s pro-innovation approach to AI comes at the cost of safe and ethical deployment of these technologies. He calls for there to be more transparency in how AI is being used in the public sector in order to better understand the risks and foster meaningful trust.
The government detailed its pro-innovation approach to AI regulation in a white paper that sets five principles of AI regulation, including safety, transparency and fairness. The paper confirmed that the government will “avoid heavy-handed legislation which could stifle innovation and take an adaptable approach to regulating.” It also stated that a new AI regulator would not be created, instead tasking existing regulators with developing more detailed guidance. Sanchez-Graells and other academics have criticised this pro-innovation approach.
Sanchez-Graells believes any light touch approach to regulation risks undermines existing safeguards around AI. “There is a lot of double-speak right now,” he says. “The white paper calls for world leading regulators and world leading rules, but with the release of the Data Protection Bill No 2, the UK government is simultaneously reducing protections for automated decision making, which is where they are seeking to deploy AI.”
CDEI recommended publicising all uses of AI in significant decisions that affect people; subsequently publishing the first algorithmic transparency standards to encourage this. However, while the recommendation was that the standard should be mandatory, the government made its use voluntary. So far, six public sector organisations have disclosed details of their AI use. But Sanchez-Graells suggests that there is more than that.
The legal charity Public Law Project has created a database called the Tracking Automated Government (TAG) register, which shows that the use of AI in the UK public sector is more widespread than official disclosures show. TAG has currently identified 42 instances of the public sector using AI. Sanchez-Graells notes the importance of these findings: “Clearly there is a big disconnect between the intent of the people working on the standard and the broader public sector.”
The cases identified by TAG are not necessarily harmful. But as the database notes: “If you don’t know that a system exists, it is impossible to know if there is an unlawful or discriminatory process at work, and you can’t challenge it to put it right.”
It is important that citizens can trust organisations to be transparent about the use of AI, explains Sanchez-Graells. “Without transparency or regulation, harmful AI uses will be more difficult to identify. This is why the pro- innovation approach to regulation, which just lets the market develop, is very dangerous.”
“This is still the tip of the iceberg,” he adds. “It’s not uncommon for governments to window dress, where they develop a standard and want to be world leading, but then nothing happens in implementation. It's not going to create meaningful trust because there is still a lack of transparency.”
There are some emerging good practices, Sanchez-Graells adds. “France has a very restrictive approach and they even have laws that forbid specific types of AI deployment in relation to the judiciary. Likewise, in Amsterdam and Helsinki, they have started to create algorithmic registers.”
Balancing the risks and benefits
Paul Maltby, Director of AI Transformation in Government at Faculty AI, says balancing the risks with the benefits is key to responsible innovation: “AI can be a powerful force for good, but it can’t be adopted widely unless it can be implemented ethically and safely. It is important that those two concepts are designed together, and that one does not outweigh the other.”
Faculty AI works with public sector organisations to embed AI safely and effectively into its processes. It worked with the NHS to forecast covid admissions and save thousands of lives, helped the London Fire Brigade target their inspections more efficiently, reduced train delays and helped the UK government combat terrorist propaganda online.
Maltby says that AI safety has become “a bit of a buzzword” but at the end of the day it is just doing good quality design. “This means thinking carefully about where these things can go wrong, thinking about where humans have to sit within a loop and ensuring that we don't automate things that shouldn't be automatable. This is really important stuff that is not always done well.”
The emergence of large language models like generative AI and chat GPT bring “a whole host of extra ethical issues,” Maltby adds. “These models have been trained on the internet, which we know is not exactly the most unbiased place.” It is important to deploy the model in a way that has checks and balances in it. There are also legal questions around copyright and IP, he notes.
“As we see this technology advance so quickly, all governments are thinking about how to mitigate the risks around this. Not just about this existential risk, but looking at how these very powerful tools are being used right here, right now,” he explains. This involves making sure that organisations have gone through a process of understanding and making a good judgement around AI. That starts by increasing education and awareness around it, he notes.
Maltby draws attention to the ideological battle happening around AI at the moment. “One side is worried about the existential risks to humanity and the other focuses on the risks AI can bring to poverty and discrimination. I find it odd that you can't be concerned about both of those things at the same time and design and regulate around them.”
Man versus machine
As part of UK Research and Innovation (UKRI), Innovate UK funds business-led innovation in all sectors across UK regions. This includes helping businesses grow through developing and deploying AI technologies
Sara El-Hanfy, Head of Artificial Intelligence (AI) and Machine Learning at Innovate UK, says they have a key role in driving ethical standards and behaviours at the earliest stages of development.
The challenge is working out how to incentivise these behaviours and translate them effectively into governance structures, she notes. “This is a complex landscape for people to navigate. Especially when the concepts of privacy, security, and fairness often work against each other. For instance, sometimes to be truly fair, you need a lot of access to certain data, which can be at odds with privacy.”
El-Hanfy says that while many initiatives exist to support businesses in establishing these best practices, there is a need for more widely available support for the AI business community. “Each sector has very different challenges. For example, if we want to put AI to work to be better at identifying rare diseases, we need AI that works better with much smaller datasets. We need to encourage more research and innovation.”
Esra Kasapoglu, Director of AI and Data Economy at Innovate UK, says the real challenge surrounding AI risk is weighing the benefits and implications of it, while working out out how to assess and manage this throughout the whole lifecycle of the AI solution - particularly when it works hand in hand with humans.
“As the saying goes, a chain is only as strong as its weakest link and the weakest link when it comes to AI is the level of people’s understanding and awareness of what AI is and their ability to deal with, and manage, unintended consequences.”
Kasapoglu says another challenge for a healthy and responsible AI ecosystem are the lingering divides between people, communities and countries on what is deemed ethical and fair. As the cycles in AI advancement get shorter, Kasapoglu says it is important to bring “everyone into the room,” including regulators, legislators, ethicists, and social scientists, to inform the discussion about the safety, security and privacy risks of this technology.
“These efforts will ensure that these technologies develop in a way that earns public trust and support innovation whilst ensuring AI is used responsibly and ethically and avoids unfair bias,” she says.
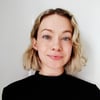